Earlier this year Motional opened up its 2023 nuPlan Planning Challenge. Fifty teams representing 11 countries responded, submitting their versions of advanced autonomous driving planners.
Motional’s team evaluated and rated the submitted planning systems using a series of increasingly challenging scenarios derived from our nuPlan dataset. The top four winners were publicly announced at the End-to-End Autonomous Driving workshop at the Computer Vision and Pattern Recognition Conference (CVPR) this past June.
The leaderboard is embedded below, following some background on the three challenges. We’ve also included a link or links to related published research.
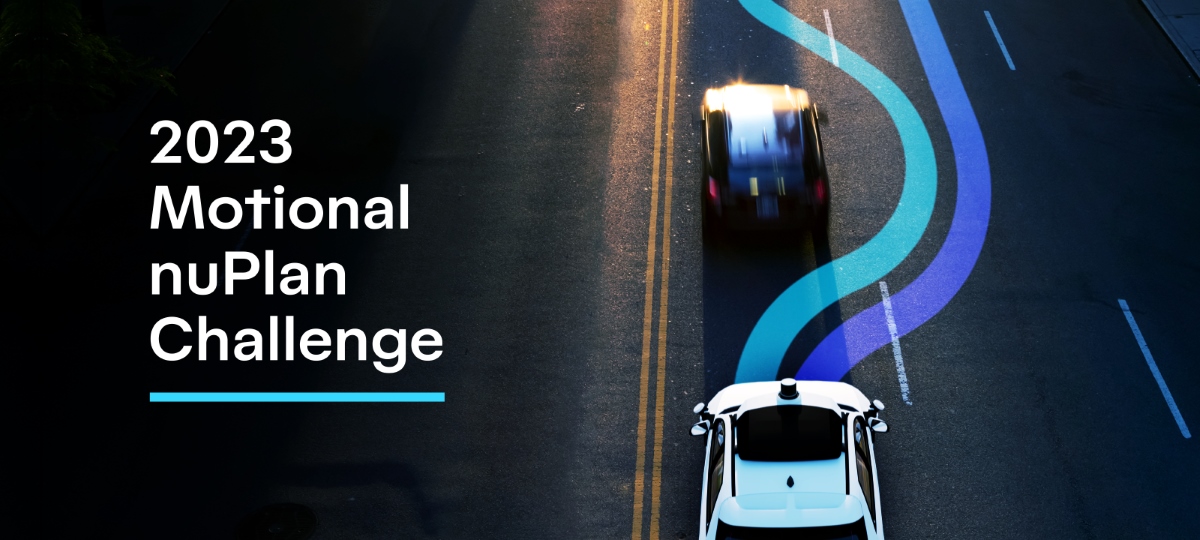
The 2023 nuPlan Planning Challenge
The main focus of the nuPlan Planning Challenge is to evaluate motion planning systems using realistic driving scenarios. In this challenge it is assumed that a planner will consume a semantic representation of detected traffic participants – such as vehicles and bicycles – static obstacles, and static/dynamic map elements with the aim to predict the vehicle’s future trajectory for a specific time horizon.
The challenge is organized into three increasingly complex modes that test a different aspect of the planners: open-loop, non-reactive closed-loop, and reactive closed-loop.
CHALLENGE 1: Open-Loop
In the open-loop challenge, the planning system is tasked with imitating the expert driver. For every time step, the planner’s predicted trajectory was scored based on a set of predefined open-loop metrics, such as the driven ground-truth trajectory.
Open-loop: Notice that the ego vehicle does not follow the planned trajectory but the expert trajectory in this mode.
CHALLENGE 2: Closed-loop, non-reactive agent
In the closed-loop non-reactive agent challenge, the planner outputs a planned trajectory using the information available at each time step, similar to the previous case. The predicted trajectory is used as a reference for a tracking controller to simulate the vehicle’s state at the next time step. Meanwhile, the other agents are replayed from a log according to their observed states in open-loop. In this challenge, planned evaluation is no longer based on the ground-truth; instead, a suite of closed-loop metrics is used to judge planner performance.
Closed-loop non-reactive agents: This mode aims to evaluate how different planners would react and perform in the exact same recorded scenarios. Closed-loop simulation allows the planner to take drastically different actions from the open-loop case. However, diverging planner actions can lead to an unrealistic simulation as shown above.
CHALLENGE 3: Closed-loop, reactive agents
In the final and most complex challenge, the vehicles in the scene react to the actions of the planner-controlled vehicle along with the behavior of other agents. An Intelligent Driver Model (IDM) policy guides the behavior of the agents. The rest of the observations are still replayed in open-loop. Similar to the closed-loop non-reactive challenge, a suite of metrics is used to judge planner performance.
Closed-loop reactive agents: With reactive agents, we avoid false collisions seen in the previous GIF. This is because each agent is now aware of other agents, but most importantly they’re aware of the ego’s changed behavior.
LEADERBOARD
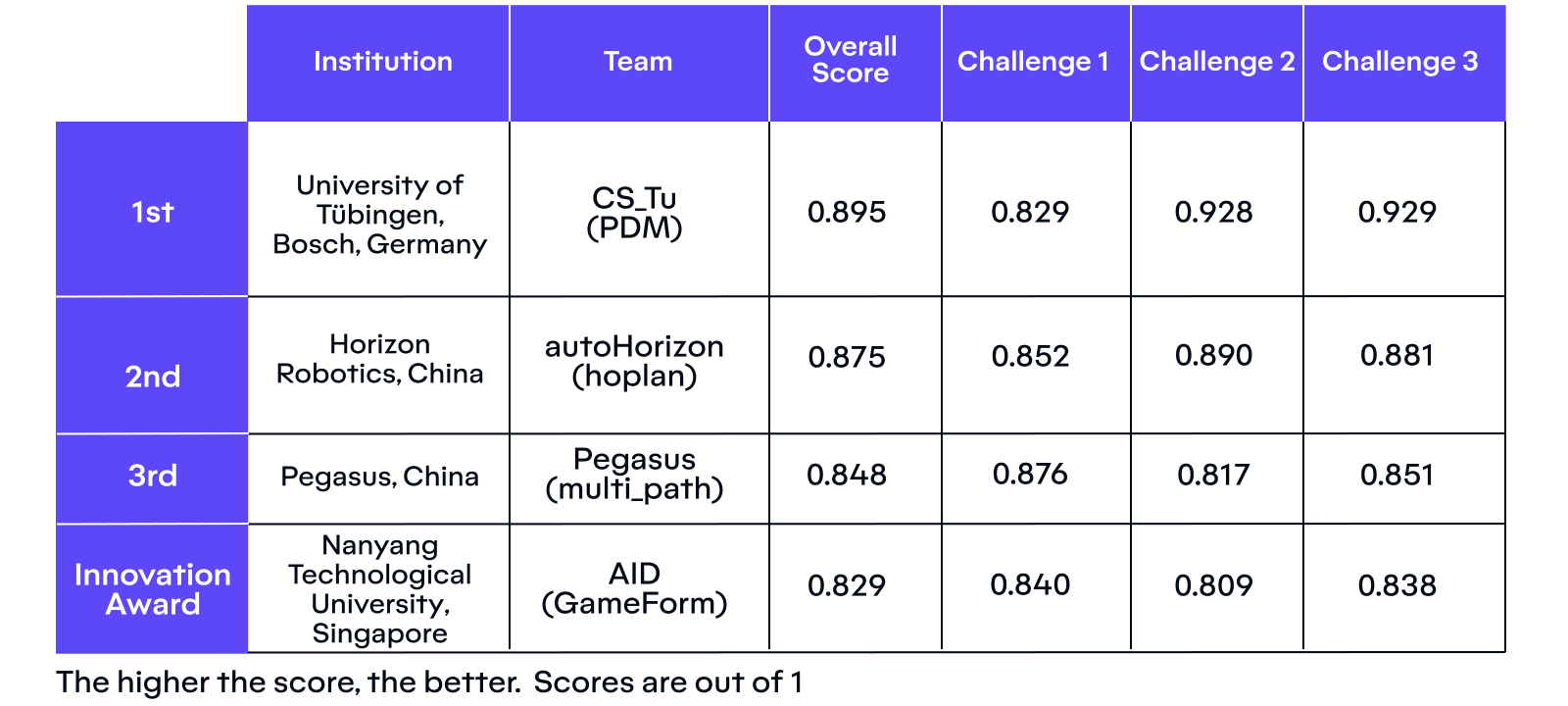
1st PLACE TEAM: CS_Tu
“We decompose the process of determining a safe and comfortable trajectory into two sub-tasks: (1) planning the short-term motion, and (2) accurately forecasting the long-term ego trajectory. While the former primarily impacts closed-loop performance, the latter is essential for the open-loop task. Our method employs a rule-based predictive planner to generate a trajectory proposal, and a learned ego-forecasting module that refines the trajectory with a particular emphasis on long-term forecasting.”
2nd PLACE TEAM: autoHorizon
“We use an imitation learning approach with several innovations, including the use of a spatial-temporal heatmap to represent the distributions of the ego's future trajectory, and a multitasking learning approach to predict and model the surrounding dynamic objects. Moreover, we fuse the predicted information with an efficient convolution algorithm to create a collision avoidance map, which is then utilized by a post-solver to plan a safe, comfortable, and feasible trajectory.”
3rd PLACE TEAM: Pegasus
“We propose an imitation learning method that incorporates data augmentation and post-processing techniques. Our model utilizes vectorized map representation, transformer encoder and decoder, and GMM output with pre-defined initial queries. In post-processing, we employ a low planning rate to mitigate error accumulation, and we use a collision detector to trigger timely replanning.”
INNOVATION PRIZE: Team AID
“We introduce the GameFormer planner, a comprehensive planning framework designed to enhance decision-making capabilities for autonomous vehicles through interactive prediction and planning. Leveraging the GameFormer model, a Transformer-based neural network infused with hierarchical game theory, our planner accurately predicts the interactive behaviors of traffic participants and generates motion trajectories for autonomous vehicles. Through comprehensive evaluations on the nuPlan benchmark, we demonstrate the competitive performance of our framework, showcasing its potential to facilitate safer, more efficient, and interactive navigation in complex simulated real-world environments.”
HYBRID METHODS SHOW PROMISE
The team at Motional would like to congratulate the prize winners for their outstanding performances! The results from the challenge demonstrated that more progress is needed before pure ML methods can compete for the top spots. Instead, hybrid methods – a combination of rule-based and learn-based methods – appears to be the most effective strategy to date.
Our thanks to all contestants for participating in the challenge. We appreciate the vested interest and continued support of nuPlan.
WE’RE HIRING!
Interested in a career working in AV technology? Motional is currently hiring for a number of roles. View open positions here!
The nuPlan dataset is free of charge for academic use, and licensing is available for commercial purposes. For more information on commercial licensing, please reach out to nuPlan@Motional.com.