
Technically Speaking
Welcome to Motional’s Technically Speaking series, where we take a deep dive into how our top team of engineers and scientists are making driverless vehicles a safe, reliable, and accessible reality.
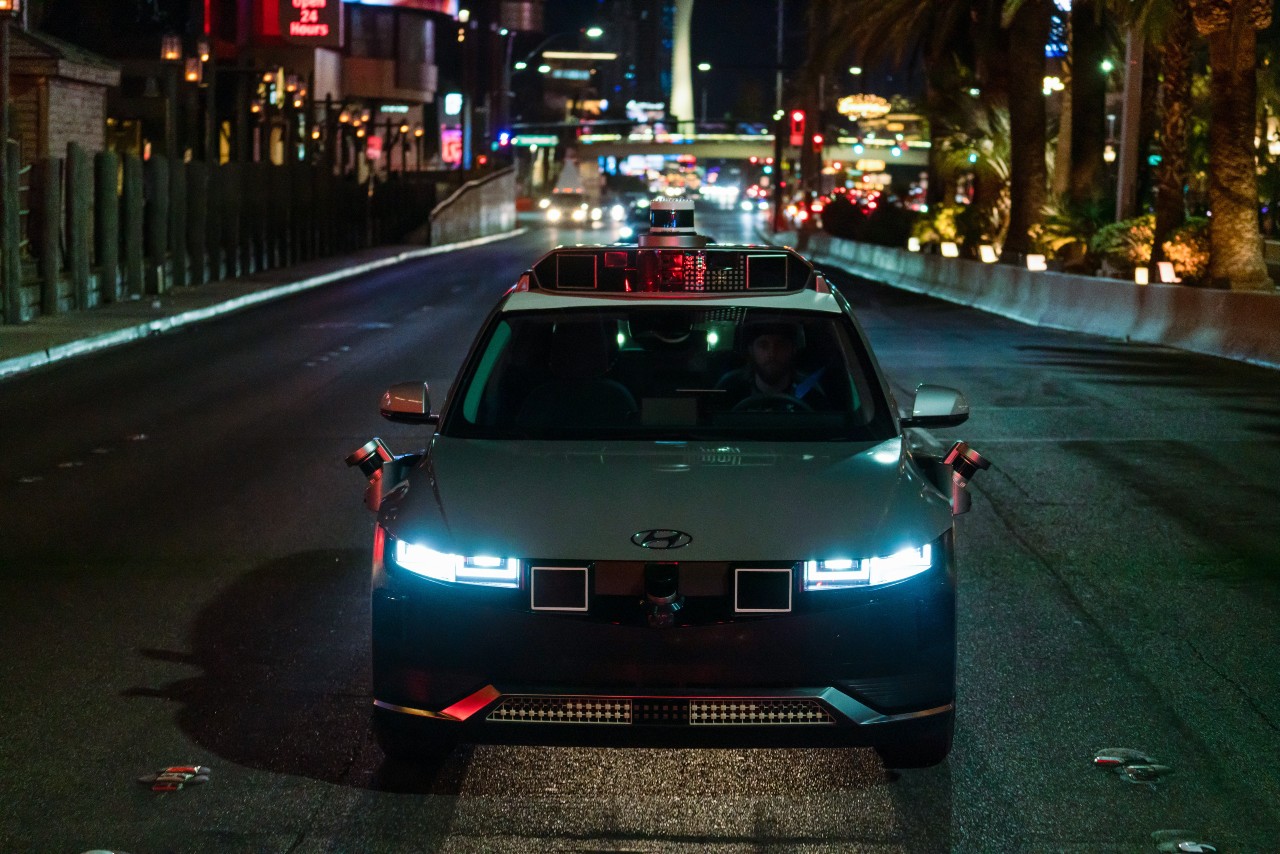
-
01 / 10
Learning With Every Mile Driven
Read MoreMotional introduces their approach to machine learning and how their Continuous Learning Framework allows them to train their autonomous vehicles faster.
-
02 / 10
Auto-Labeling With Offline Perception
Read MoreMotional shares how they build a world-class offline perception system to automatically label the data that will train their next-generation vehicles.
-
03 / 10
nuPlan Dataset Will Advance AV Planning Research
Read MoreMotional's expanded open-source planning dataset will allow our industry and researchers to better understand how a driverless vehicle can find its way through a dynamic environment full of obstacles and ever-changing circumstances.
-
04 / 10
Mining For Scenarios To Help Better Train Our AVs
Read MoreMotional is using AI to sift through mountains of vehicle data to find the unique driving scenarios needed to make AV tech smarter.
-
05 / 10
Predicting the Future in Real Time
Read MoreHenggang Cui explains Motional’s approach to prediction, and how we use multimodal prediction models to help reduce the unpredictability of human drivers.
-
06 / 10
Traveling Back in Time to Help AVs Plan Better
Read MoreCaglayan Dicle takes a look at how closed-loop testing will strengthen Motional's planning function, making the ride safer and more comfortable for passengers.
-
07 / 10
Using Machine Learning to Map Roadways Faster
Read MoreThis article explains how we're using machine learning to speed up the process of mapping public roadways prior to launching commercial passenger service.
-
08 / 10
Improving AV Perception Through Transformative ML
Read MoreMotional is using Transformer Neural Networks to improve AV perception performance.
-
09 / 10
How Continuous Fuzzing Secures Software
Read MoreMotional employs a technique known as fuzz testing, or fuzzing, which stress tests their autonomous vehicle software through the use of randomized, arbitrary, or unexpected inputs.
-
10 / 10
Improving Multi-task Agent Behavior Prediction
Read MoreMotional introduces PredictNet, a prediction module that gleans insight from past incidents to better predict the behavior of vehicles, people, and other agents in the driving environment, thereby increasing safety.
Curious about our technology?
Learn more here.